Home > Resources > Newsletters > The AI Vue
5th December 2024
Wait, my AI has an MBTI?
The priority should not be chasing the latest trend but solving problems effectively. In the rush to embrace Generative AI, it’s easy to overlook the vast array of other powerful AI techniques available.
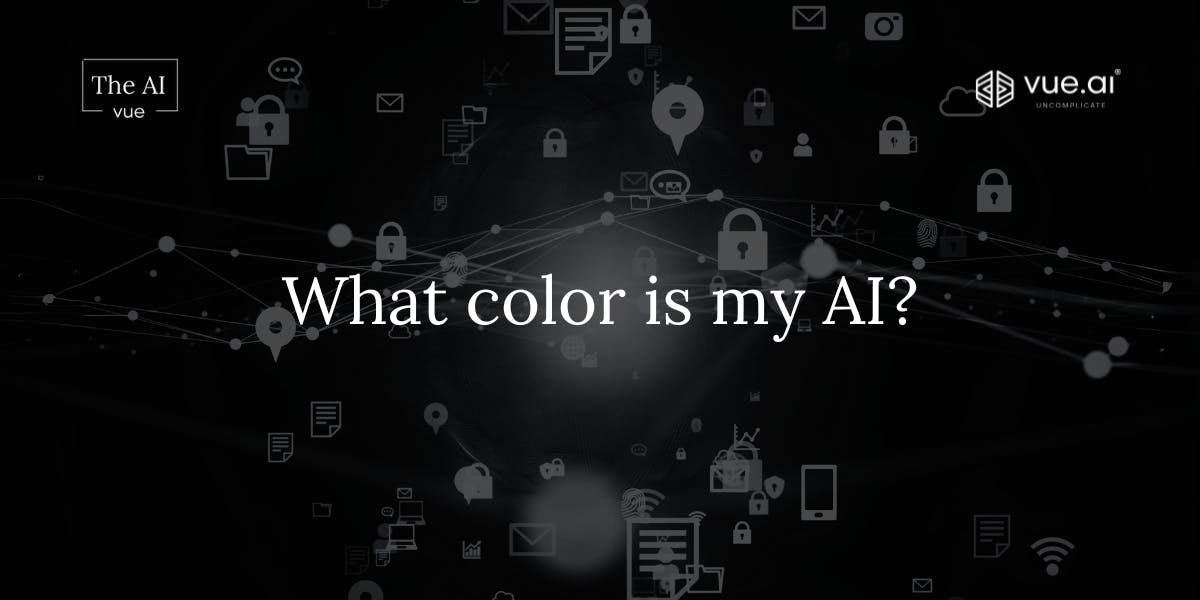
AI evokes a sense of wonder, offering the promise of transformation and boundless innovation. Yet, this excitement must be tempered with careful consideration of its limitations and an understanding of the challenges it brings. The allure of shiny new technologies is hard to resist. Generative AI (GenAI), in particular, has captured imaginations and boardrooms alike, emerging as one of the most talked-about innovations in recent years. Gartner even released its second Hype Cycle for Generative AI earlier this year, cementing its position as the technology du jour. But as messianic as it may seem, GenAI is hardly a one-size-fits-all solution.
For business and technology leaders, the question isn’t how to implement GenAI, but rather, how to solve business problems using the most effective AI tools available. AI is not a monolith; it spans a wide range of techniques, each suited to solving different types of business problems. Different use cases benefit more from particular AI techniques that are more reliable, efficient, and tailored to specific business needs, and many others benefit from no AI at all. The key is to approach AI strategically, leveraging the full spectrum of available techniques—and, where possible, combining them for maximum impact.
Below is a primer on a few that have yielded remarkable results.
1. Non-generative Machine Learning (ML): The classic workhorse
Core: The cornerstone of AI applications for over a decade, this form of AI uses historical data to make forecasts and predictions. Alias: Predictive ML.
Contrast: Compared to GenAI, predictive ML is often faster, more cost-effective, and better suited for structured problems with clear outputs.
Clout: Thrives in scenarios where accuracy and precision are paramount. Excels in use cases like customer segmentation, anomaly detection, churn prediction, and maintenance forecasting. Can power recommendation engines, offering personalized suggestions based on a user’s past behavior. (Watch: How Tata Neu cracked the code on user engagement and Watch: How Sage Parts improved customer experience by optimizing their catalog)
2. Optimization: Balancing trade-offs for complex decisions
Core: Optimization techniques are the unsung heroes of operational efficiency. These methods evaluate trade-offs to find the best allocation of resources under given constraints.
Contrast: Unlike GenAI, which generates content or insights, optimization directly supports decision-making processes for tasks.
Clout: Critical tool for businesses looking to streamline operations while balancing competing objectives: Route planning, workforce scheduling, inventory management, and financial portfolio optimization. (Watch: How Buyma uses Vue.ai for inventory management)
3. Simulation: A virtual testing ground
Core: Lets businesses test ideas, strategies, or changes without taking on real-world risks. Can also produce synthetic data to train other AI models. (Watch: PICARD profits from Vue.ai’s solutioning)
Contrast: Provides a controlled and explainable alternative to GenAI.
Clout: Especially valuable for long-term planning and “what if” scenario analysis. Used in supply chain planning, financial modeling, workforce planning, and even training autonomous vehicles.
4. Rule-based systems: Knowledge in action
Core: Encode human expertise into structured rules for decision-making. Alias: Expert systems
Contrast: Unlike black-box GenAI models, rule-based systems are easy to interpret, making them ideal for industries such as finance and healthcare that demand explainable outcomes.
Clout: Transparency is their trump card. In areas like fraud detection, loan approvals, medical diagnoses, and quality control, they triumph. (Watch: Cataloging 250 million used garments–one of each–using AI)
5. Knowledge graphs: Mapping relationships for precision
Core: Offer a structured way to retrieve precise information, by organizing data into networks of nodes and relationships.
Contrast: When combined with GenAI, they add an additional layer of factual accuracy, ensuring that the generated outputs are grounded in verified data. Makes them an excellent tool for businesses that prioritize reliability and trustworthiness.
Clout: Shine in applications like search engines, recommendation systems, and fraud detection. (Watch: Dubai CommerCity’s transformation to paperless operations)
o- - - - - - - - - - - - - - - - - - - - - - - - - - - - - - -o
Read other editions of BFSI Vue
The Wolf of Invoice Street (Nov '24)
Credit needn’t chase its tail (Oct ‘24)
AI and APIs in insurance land (Aug ‘24)
o- - - - - - - - - - - - - - - - - - - - - - - - - - - - - - -o
The case against GenAI as the default solution
Generative AI has undeniable strengths, such as generating creative content, synthesizing text, or summarizing large datasets. However, it comes with limitations: lower explainability, higher computational costs, and a tendency to generate outputs that may lack precision or reliability (Read The degeneration of Generative AI). For use cases requiring stringent accuracy or transparency, simpler AI techniques are often a better fit. Starting with simpler AI approaches often reduces risks, costs, and complexity. These techniques are typically easier to implement, understand, and maintain.
The power of combining techniques
The most sophisticated AI systems don’t rely on a single technique—they combine multiple methods to leverage their strengths. For example:
- Pairing simulation models with optimization techniques to test and refine resource allocation strategies.
- Enhancing knowledge graphs with predictive ML for more targeted recommendations.
- Using GenAI to create initial data inputs, which are then refined and validated by rule-based systems or optimization algorithms.
This blended approach delivers not only better accuracy and performance but also greater flexibility and resilience.
Next is what?
To enterprise leaders looking for ideas in their AI transformation plans:
- Start with the problem, not the technology: Define the business problem clearly and understand its unique requirements. Only then evaluate which AI techniques align best with your needs.
- Experiment and iterate: Begin with simpler AI methods to gain quick wins, and scale up to more complex systems as needed.
- Invest in a modular AI strategy: Build systems that can integrate multiple AI techniques, enabling flexibility and future-proofing your investments.
- Focus on explainability: For high-stakes applications, prioritize techniques that provide transparency and accountability.
- Combine, don’t compete: Adopt a mindset of collaboration between AI techniques, leveraging their complementary strengths.
And, so…
In the rush to embrace Generative AI, it’s easy to overlook the vast array of other powerful AI techniques available. For business and technology leaders, the priority should not be chasing the latest trend but solving problems effectively. By adopting a thoughtful, diversified approach to AI, organizations can achieve superior results—delivering better accuracy, transparency, and cost efficiency while building a sustainable foundation for future innovation.
Getting ideas? We’re happy to bounce off. Two brains are better than one—even if one of ‘em is artificial.
About: Vue.ai is an AI orchestration platform that actively caters to enterprises in retail, BFSI, logistics, healthcare and other domains. Deploying a mixture of expertise in various AI techniques and on platforms like AWS, Azure and GCP, Vue.ai charts productive digital transformation journeys for clients across continents.
o- - - - - - - - - - - - - - - - - - - - - - - - - - - - - - -o
Also read The AI Vue
Smart AI still needs human eyes (Nov '24)
Agents are here → AI’s shaken and stirred (Sep '24)
Lifting the lid on LLMs (Aug '24)
o- - - - - - - - - - - - - - - - - - - - - - - - - - - - - - -o

- Enterprises deploy too many single-purpose applications that cannot easily communicate with each other in their workflow, creating a disjointed system.
- An overabundance of data science and machine learning tools makes it challenging to maintain models efficiently, leading to high operating costs. Creates tension between data- and model-centric approaches.
- Massive economic costs associated with broken data cost organizations trillions in unnecessary losses. Who'd want to be a data janitor, huh!

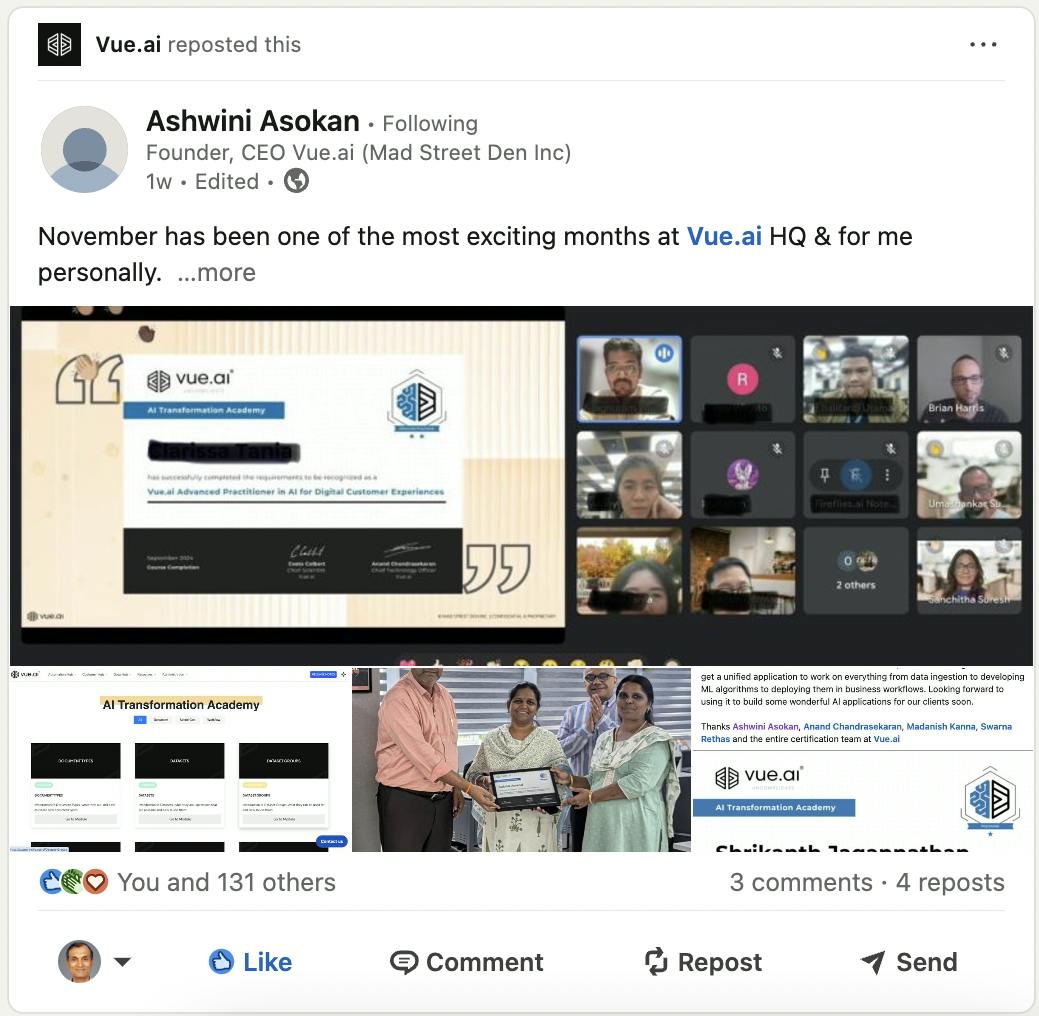