Home > Resources > Newsletters > The AI Vue
7th November 2024
Smart AI still needs human eyes
The aim of AI automation systems might well be to get the human out of the loop; but it is the human who is very much in the loop at this phase of AI development, whose role is crucial in ensuring business outcomes.
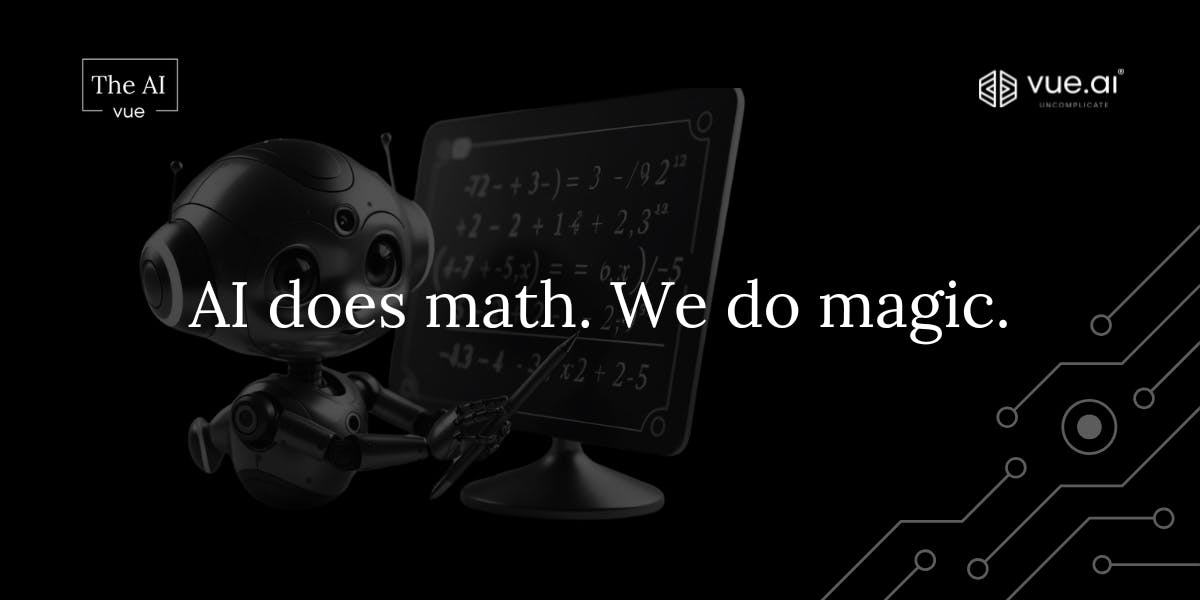
The promise of AI is both exciting and concerning, in a rapidly growing range of industries. From unreliable answers to ethical missteps, the challenges and risks of AI make it clear that human oversight is not just beneficial but essential. HAL. Sonny. Chitti, even; human control over machines has been necessary even in fiction. Optimizing the synergy between AI systems and human insight to drive reliable, responsible business outcomes is the way forward.
AI in enterprise - Risks and realities
The role of technology has always been that of an enabler, a supplement, so to speak, for humans in order that they move on to higher pursuits, leading machines to enact the moribund life. While AI has proven capable in many fields, its adoption is not without significant challenges. A recent example is UPS. With plans to leverage AI for efficiency gains, the company is simultaneously facing job cuts and reduced revenue, largely driven by lower package-delivery volumes and missed earnings targets. AI alone can't address these complexities or serve as a cure-all for business hurdles. For UPS and others, AI should augment human roles rather than replace them outright, especially where context, judgment, and adaptability are critical.
Adding to the list of examples, Air Canada encountered issues with its customer service chatbot, which misinformed customers on discounts. When the case was brought before a tribunal, the airline’s defense—that the bot was an independent legal entity—failed. This incident underscores that for customer-facing AI, quality assurance and accountability are paramount. Companies cannot treat AI as a "set-it-and-forget-it" solution; regular human review and calibration are essential for systems that directly impact customers. (read Horns or halos: The true nature of AI)
Balancing autonomy and accuracy
While AI has shown success in various tasks, such as Dutch lender ING Groep NV's adoption of reinforcement learning to automate currency pricing, this technology works best when deployed with clear boundaries and regular oversight. ING’s AI model efficiently adjusts spreads and manages risk, yet relies on reinforcement learning, a process that closely mimics human trial-and-error learning. This approach performs well in stable, repetitive tasks but could falter in more nuanced scenarios where human traders’ judgment is required.
In military applications, human oversight remains even more critical. For instance, the U.S. military’s Project Maven, which leverages AI to identify targets, revealed AI’s stark limitations. AI identified military objects with a mere 60% accuracy, compared to humans’ 84% success rate. In challenging environments, like snowy or low-visibility conditions, AI’s performance dropped as low as 30%. Will Roper, one of the project’s leads, aptly observed that AI should be considered more like a “junior officer”—one that requires supervision, guidance, and clear operational boundaries.
These examples illustrate a broader insight: while AI can assist with repetitive tasks, it lacks the logical reasoning required for high-stakes decisions, making it necessary for human operators to ensure that AI outputs align with business and ethical standards. (also see Your victories are my celebrations, says AI to humans).
Specialized and supervised AI for optimal performance
In recent years, some industries have begun to recognize that “one-size-fits-all” AI models are more prone to error and bias. Specialized AI applications, tailored for specific domains, show promise in reducing biases and increasing accuracy. One technique, Retrieval-Augmented Generation (RAG), attempts to refine general-purpose AI models, adding more contextual accuracy by referencing external databases. Yet, RAG itself is insufficient for reasoning-based tasks, as it lacks a formalized logic or “guardrails” to ensure precision. AI today still struggles with interpreting nuanced data, which can result in errors like the ones Stanford University found in its legal tests, where AI models only achieved a 25% accuracy rate on case-law questions.
To offset these issues, tech companies and researchers are exploring more layered approaches, including using AI to monitor other AI systems. Known as "Foundation Model Operations" (FMOps), these tools are part of an emerging AI quality control practice, testing and evaluating outputs in real time. By using FMOps, companies can adapt and customize models while preserving accuracy, but they also reinforce the need for executive-level investment in AI oversight.
Takeaways for tech and business leaders
- Invest in monitoring and accountability: AI has clear limitations that make regular monitoring essential. Whether it’s customer service, trading, or other critical operations, implementing FMOps tools can provide ongoing quality control, reducing risk and increasing AI reliability.
- Deploy AI for routine, not critical decisions: AI is effective at handling repetitive, rule-based tasks. In complex, context-sensitive areas, human judgment remains irreplaceable, especially when facing unpredictable scenarios.
- Prioritize specialized models over general solutions: Industry-specific models reduce biases and increase relevance, as seen in the financial and healthcare sectors. For companies venturing into AI, custom AI systems can yield better results than generic models trained on broader datasets.
- Support AI training with clear guardrails: AI lacks a built-in logical framework, so setting precise rules and limitations for its use is crucial. Employ formalized rules to guide AI decisions, minimizing the risk of errors and promoting more consistent outputs.
Better together for breakthroughs
AI, by definition, is probabilistic and not deterministic. The aim of AI automation systems might well be to get the human out of the loop; but it is the human who is very much in the loop at this phase of AI development, whose role is crucial in ensuring business outcomes. As AI’s capabilities grow, its greatest asset is not in replacing human roles but in enhancing them. With the right balance of autonomy and oversight, AI can deliver significant value without compromising quality or accountability. Embrace AI not as an autonomous force, but as a strategic partner that benefits from human experience and supervision. By keeping AI accountable and focusing on synergy, businesses can harness its strengths while mitigating risks, positioning AI as a responsible, effective tool in the enterprise.
< - < - < - < - < - < - < - < - < - < - < - < - < - < - <
Read other editions
Agents are here → AI’s shaken and stirred (Sep '24)
Lifting the lid on LLMs (Aug '24)
See through Computer Vision (Sep '23)
> - > - > - > - > - > - > - > - > - > - > - > - > - > - >

When green means go wrong: a camping tent for avocados? A quirky error only automation could produce!

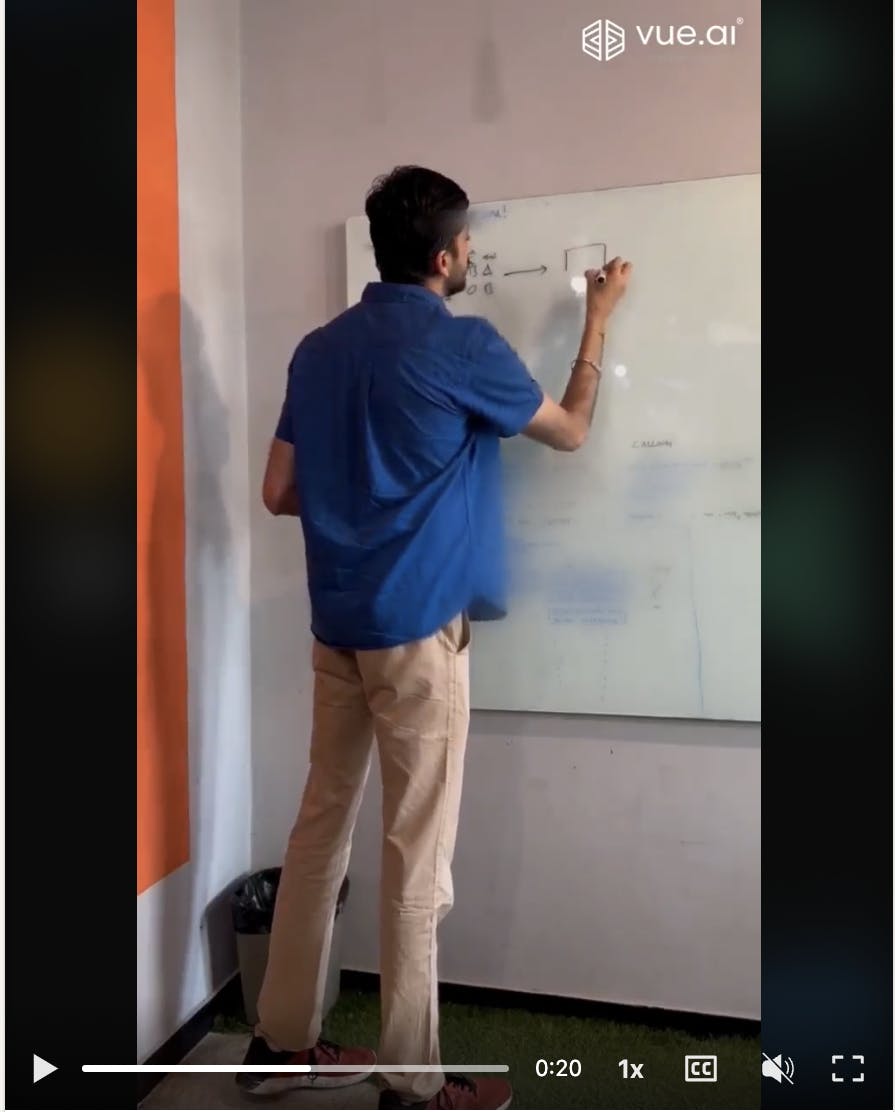
Behind the scenes with our ML engineer training our star performer Dial in.