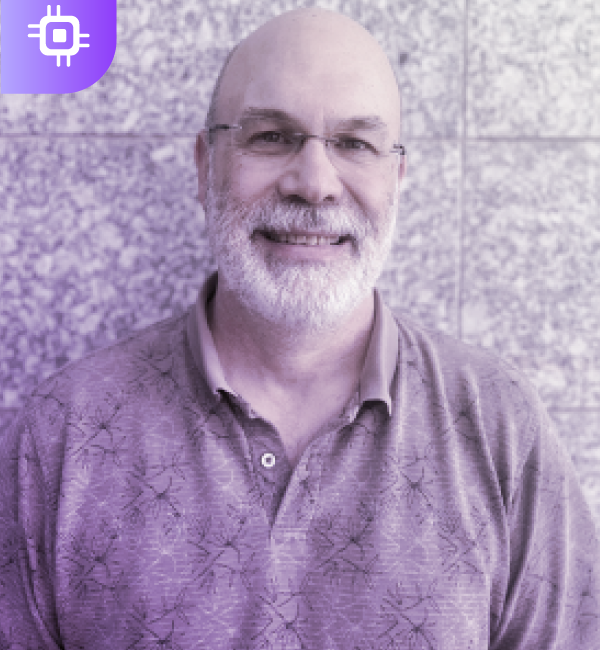
A Chat(GPT) with Dr. Costa Colbert
About this Podcast:
The AI Transformation Podcast
A Chat(GPT) with Dr. Costa Colbert
Welcome to the first episode of The AI Transformation Podcast! To start things off, we sit down for a Chat(GPT) with Costa Colbert, Co-founder & Chief Scientist, Mad Street Den, to discuss all things generative AI – the hype, the use cases, the facts, and how it plays against the fiction. Does it really deserve the hype? Are these systems really intelligent? What are the themes emerging from generative AI? This, and more!
Costa holds MD and PhD degrees in neuroscience from the University of Virginia along with biomedical engineering, electrical engineering and computer science degrees from Johns Hopkins University. He is well-known internationally as an experimental and computational neuroscientist in the area of single-neuron electrophysiology. He has been the principal investigator on grants from the National Institutes of Health and others to study how neurons encode and transmit information. After taking the leap from academia to industry, he built perhaps the first large-scale GPU-based neuron simulator, long before GPU’s were widely used in machine learning. Today, he is the Chief Scientist at Mad Street Den, building advanced neural network architectures that can enable more generalizable models of intelligence.
Listen on:
Episode Transcript:
Hey there. Welcome to the AI Transformation podcast by Team Mad Street Den. I’m Shyam, and I host this podcast with my founder, Ashwini Ashokan. At MSD, we've been bringing together leaders from across the world - CIOs, CEOs, digital transformation heads, CTOs, product and marketing leaders who have been crucial to transforming their businesses with AI. Today I have with me Ashwini in conversation with Costa Colbert, our co-founder, and Chief Scientist at MSD. Costa was a professor of neuroscience and has an impressive list of patents and innovations on the topic we're about to discuss today. ‘All things generative AI’ - who better than Costa to help us understand fact vs fiction surrounding what's going on in the market today, with ChatGPT, Stable Diffusion, and the world of Generative AI. Welcome, Costa and Ashwini.
Ashwini AsokanAlright, Costa, so excited to have you on board and I know that we've all been waiting for this conversation with you, given all things generative AI is blowing up. So I wanted to start off by basically asking you, what's with the hype? What's going on? What's with all the excitement around the news we're seeing? Just give us a little bit of color on all things generative AI, at least as it relates to the noise in the market. Before we can go in there and start picking out what's real, and what's not real, what could we do with all of this that's that we've been seeing in the market? I would love to just start off by understanding what's going on. What's with the hype?
CostaWell, I think the biggest reason for the hype is that the latest round in the last year or two of what falls into large language models and also image generation, which are two things that are actually quite connected, is sort of all things to all people. And so it's hard to find somebody who doesn't have something that they do that they'd like an AI to help with. And the things like these large language models start to start to give people the feeling that they might be able to do anything they want. The idea from science fiction that we will eventually have AI that either helps humanity or dooms humanity. You know, we're getting closer to where that feels like feels like a reality and less like a science fiction thing, even though it probably is still quite science fiction. It does start to feel more real.
Ashwini AsokanOkay. So I feel like there's a little bit of a wow quotient here, like when you start to see some of those images being generated. They're beautiful colors. It's this, it feels it has almost like this art. Like really, really complex and very cool art quality to it, especially on the visual side of things. Right? It's a generic space. It's not specific in a lot of ways, but there is this, almost like this glamour, a very glamorous, very beautiful feel to what's being created, which I think is also causing some of that almost visceral reaction to all of that.
CostaYeah, I think it even goes broader than just beautiful images. You also have some very, very real-looking images. You know, you tell one of these systems you want photorealistic and, in some cases, does a fine job of making something, at least at first blush, look photorealistic. So I think that's part of it. I think that you can, you know, and the other thing, too, is, again, in the sense of all things to all people, you take this one system, and you say, draw me a car or draw me a dog or draw a beautiful landscape or something. It's not specific to one small domain. It's just whatever you want. It seems to be able to do.
Ashwini AsokanYou know, something that.. it feels like the Alexa and the Siri days. When Siri first came out, I mean like kids were obsessed with like asking Siri this and asking Siri that. And we saw the same thing happen with Alexa. And more and more people were just experimenting with, Do you know this? Do you know that? Are you smart? Are you this? And I think we've seen a little bit of that fading over time as well, because people are like “Alexa, Alexa” and so mad. She just keeps coming back with, “I don't understand what you're saying…”
CostaThat's right.
Ashwini AsokanThat's right. So it's almost feeling a bit like that, that level of hype and excitement right now.
CostaThat's right. That's right. I think I think you're going to run against the wall with these models. It's not going to be as easy to run against the wall with these models. There are many orders of magnitude more, more capable and in what they're going to do - before, they basically just say, and in fact, that may be one of the problems that they don't say they don't know. They just give you an answer that they just made up. And so we may not even know that they didn't know. But I think it is exactly the same type of cycle, and it's exactly the same type of thing where you look at some of what is generated, and it's fantastic. I have a friend of mine who is a professor who said that one of his colleagues was very upset that it was capable of writing papers that he would say would be, let's say, a B paper, at a university for a particular subject. Maybe that's a little bit optimistic, but the idea was, it was good. And what my friend came back with was, “I don't know, if it comes back to me with clear writing and topic sentences and proper paragraph structure. I'm okay reading that.”, So yeah, funny that in a lot of the mechanical sort of ways that are not trivial by any means at all. They're very, very capable systems, and they can generate prose, and they can generate poetry, and in all of these sorts of things in a way that is pretty good. And a lot of people are not good at that. So if you're not really comparing, you know, the poem that the AI wrote compared to the poem that a well-respected poet wrote, then there's one comparison. But if you say write a poem against a first-year university student, it may do much better. And that's actually an accomplishment. So that in that sense, I think that some of the hype is actually very well deserved.
Ashwini AsokanWhat are the kinds of use cases, and applications that are possible? Right. Both on the language side as well as the image side. What's a reasonable set of expectations around what's possible?
CostaYeah. So, in thinking about use cases, one of the very important issues that's not been completely understood at this point is what is it that these models can and can't do as a function of the way that they're built, and what are the things the models can or cannot do because of the nature of the training data that you need or that they've been trained with? And sometimes, those are very difficult to tell where the problem lies. Anything. What seems to be pretty clear, given the nature of how these models work, So a model that's something like and there are others, but a model like GPT, which is ‘Generative Pre-trained Transformer’, their basic idea is to be able to take a prompt of words and be able to just predict what the word should be that should come afterwards. And when we think about interacting with something like Chat GPT, which really does seem, at first, it does seem like there's a person on the other end typing back. You wouldn't necessarily realize that that's what it's doing, but that's the way that it is built. So you, you give it a prompt, and then it's basically figuring out probabilities of what should be next.
CostaSo thinking about it in terms of the Eliza program, it's in a sense doing the same type of thing, except many, many orders of magnitude more powerful in this. But it's still…you could think of it as being an incredible pattern-matching machine and template-matching machine. So anything that is a syntactic manipulation, so anything that's like a translation. So it could be from translating between two human languages, or it could be translating between a, let's say, English and then some computer programming language. That's one of the things that's really hot right now is that you can say to this thing, write me a program in this particular language that does such and such, and it does a remarkably good job a lot of the time for a lot of the pieces. And then you can even say, explain it and explain why you said do this part or that part, and it will give you more text that's doing the explanation. But it's still sort of a big template match. There isn't really any reasoning that has to go on there.
Ashwini AsokanRight. I guess, to some extent, it's also just because of how large the vocabulary is, right? That really is, vocabulary, meaning just how much data has gone into this, right?
CostaYes, that's exactly right. That's why it's difficult to tell sometimes. And I saw that somebody had mentioned in a very good way that one of the things that these language models show us is how many different things that we do actually don't require any intelligence. And what they mean by that - definition of intelligence is actually kind of tough - and what they mean by that is the definition of intelligence is actually getting tougher because a lot of the things that you would assume at the beginning you need some intelligence for, don't really need that much intelligence. And in the sense of coming up with something that's really novel or coming up with something that requires some kind of complex reasoning. And that's probably the biggest thing. It's just that it's hard to tell that the system is not reasoning when in fact, it really is able to accomplish what you think is reasoning by this very, very complicated pattern matching that's able to happen. So going back to the idea of use cases, then the question is, if you have a particular use case, does it fall under one of those sorts of categories? And the reality of it is that many, many use cases probably can fall under that category. And so that's probably why part of going back to the beginning of our discussion, part of why it's so exciting is so many people can see value and in all sorts of different areas of how you can use this.
CostaThe problem starts to come in, though and related to this is let's say that you want to use language model to generate… Let's say, you need to write a newspaper story. So let's say you decide that you want to be able to write, to have this system, write you a quick paragraph for a news story. And so you want to be able to put in a prompt that looks something like a tweet that has the meat of the matter in there. And then it's going to generate this copy for you. And you can even go further and say, for whatever reason, you could say a style if and if it's been trained with enough data that it can associate a particular style with some of the text that it's seen. You can say something like, you know, write this copy and in the style of Winston Churchill. And so you could get this very pompous paragraph out of it, something like that. Yeah. So let's say it writes to the copy, it reads pretty well, but maybe it has some statements in there that are either totally irrelevant to the story or they're just factually wrong. So you as the copy editor, can say, other than this one sentence, this is good to go. I edit the one sentence I go on my way. This has provided value for this case because it did something.
Ashwini AsokanLike the role change of writer versus editor, right?
CostaYeah. And in the larger picture, the point that I'm getting at is really that at least in January of 2023, and given the state of these models, is that you pretty much can't assume that the output that it gives you is going to be entirely correct. And so somebody has to vet it. And if you're in a situation where you're expecting to vet it, there's no reason you would send copy out without somebody actually reading it and checking what's there. Then it's sort of a natural fit, and it may have a lot of value. Conversely, if you have a use case where you think it could actually work pretty well, let's say you wanted to have a website that ingests tweets and generates paragraphs that have more context. So, somebody that might not know what's going on in this thread of tweets, you can read it, and you want this thing just to be running 24/7 unattended. That would probably be a recipe for disaster because you don't actually have somebody editing and reading and making sure that these things are correct. So I think that's, you know, that ends up being a lot of what the use case is now there because there's so many people out there. I mean, probably tens, if not hundreds of thousands of people now who are looking at this. You have an army of people trying to think, what can this do for me? And of course, coming up with all kinds of creative ideas.
CostaAnd I know that if we extend this a little bit and talk about as well, not just the text, but think about pictures, then you could imagine use cases where somebody says, let's come up with an ad campaign and I want to see a few sample images of what might be a nice, you know, a nice background or a nice way of presenting something, then in a few seconds, you can generate a whole bunch of images and you could say, “Oh, I like the style of this one, or I like this, or, Oh, this gives me an idea about that”, And depending on the prompts, you might be able to to go in a particular direction and make some interesting pictures. And if you had sent it out to an artist just to start to give you some ideas, maybe that turnaround time is a week or two. And so that could be very good as well. So that use case could be fantastic. The problem you might run into is when either the art director or whoever's the art director is boss says, “Oh, that's exactly what I wanted. Let's use it.” And then, you find out that the baby in the image has three fingers that are very long and looked like it's an alien baby. That's kind of a funny example, but the reason I thought of it is because I've generated pictures where that's. That's what they look like.
Ashwini AsokanI almost want to just dwell a little bit on this fact versus fiction. What is fact here? What is fiction here? Where does this break? Where does this work really well?
CostaSo the fact is that there's a tremendous amount of information built into these systems, but they don't have all the information. And they also have a tendency to encode whatever biases and incorrect information. So, for example, some of these language models were trained with Wikipedia and everyone knows that Wikipedia has fantastic pages and terrible pages. And it really depends on which page. Well, if you indiscriminately pass all of these pages through this model, then you ask a question that is.. aligns very well with something that's on a Wikipedia page, then it's going to generate information that's wrong. So the idea that you can basically trust anything that comes out of the model is basically thrown to the side. And so if you can vet what's there, you're good. If you can't vet what's there, then you have a problem, as we said before. So another thing is that it appears that when you look at this thing, it appears to be this oracle that seems to know everything about everything. And that's because it's been fed a tremendous number of documents. There's one model that I was looking at recently where they were doing they were looking at pairs of images and short bits of text to describe what's in the images as the training data.
CostaAnd they used 400 million pairings. It's a tremendous amount of data. And if you think so, even if cost was no object, and of course it is, and that's an issue. If cost was no object, if somebody asks you to collect 4000 examples, then think how much, how long that would take you to do. And yet 400 million. So if you're doing 400 million, there's no humanly way possible to vet the information that's going through. Right. It also means that it's still incomplete as much as you're reading all this. So one bit of sort of fact versus fiction that that maybe is not quite so obvious is it seems to know everything about everything, but there's probably less information in there than if you were to do a Google search. And so, if you do a Google search, the correct answer might be, and what you're looking for might be on page ten. But it might actually be there. Whereas the same way that the stuff that's on the first couple of links that the Google search gives you might be bad. That might be what the language model is just going to serve up as the answer.
Ashwini AsokanIt almost feels like, you know, if we had to go down what is fact, what is fiction here, especially in the language side, I would love to move the conversation down to the visual side of things here. But it really is beginning to feel like, on one hand, it really is a question of accuracy, right? Like how much of what is being said and generated out there is accurate. And so having people in the loop to be able to verify the accuracy or redo some of that. So there's a bit in the accuracy part of it, right? The other part of it that we've also seen is that the more you keep doing it, the more all the writing starts to look similar. And the more and more you do it, and we've all tried this enough number of times, it almost feels like it's all merging, right? There's a bit of everything beginning to look the same kind of a feeling that's happening, which is still fine because I do think all of us have completely different applications or use cases for it. But it is important to understand that within the business, for example, generating descriptions for different types of products or different types of inventory and merchandise that we deal with when we're talking about applying AI in production in scale for retail. And it cannot look the same. And because people are out there, they're searching for stuff, and there's SEO involved. You're looking to get a very accurate description of what's going on with that piece of clothing or with that product and you do not have the luxury of all of these things merging to look the same rather than having... So there's that part of it as well.
CostaLet's say you're trying to help have it help you write a novel, and you say, okay, the first paragraph is good, the second paragraph is good. I don't really like this one that much. Let me try that again. So there has to be an ability there to have some variability, right? So variability is good for what we just said, but it's bad if there's a correct answer, it's bad if what you're trying to generate is supposed to be a particular answer. So anything in terms of use case that falls into something where the correct answer actually where there is a correct answer. Right. So there was a.. actually, I think you said it to me, there was a little Twitter thread that was talking about somebody had a list of references for a paper, and they wanted it to be formatted. And they were using a particular system that's known to be very finicky in how you format it. So he said he had ChatGPT format these references in the style of this particular [way of] what this program wanted, and it did it and he was thrilled because he had this very tedious work done for it, so people started trying it a little bit more and, and, and it was generally working.
CostaBut the one most interesting thing here is that the system started changing the names of the titles and the authors, not all the time, but it started changing. And you could imagine that there are some dials and knobs, and there's a way that maybe you can decrease for that particular thing. You can decrease the amount of variability, but the system is built to be variable and built to be having different answers. And so something as simple as a template match where you're saying these are this is the correct answer that I want. I don't want a different author on this paper. I want the right author on the paper is a very good example of a failure mode for this type of system and any kind of use case revolving around that, you would have to think about it.
Ashwini AsokanIt's almost like there's, I mean, and the way I see it as I've been seeing all of these examples, and it feels like there's two types of themes emerging, right? There's the theme around, I want to get a job done, I'm looking to essentially not do that work, and I need the system to do it for me. Which is one class of applications, right? That is the intent behind using something like strategy or and then there's another side of it, which is all around exploration, art, and play. It feels like play is the theme there, where accuracy is almost not important. And so it's okay to have a three-fingered alien baby or, you know, it's fine, right, to have almost like a factually incorrect. In some cases the physics is wrong. In some cases, all of that is fine because it's play, it falls under the bucket of art. And so accuracy is a lot less important. And the other category of..s it's interesting to see that, I think one of the biggest themes emerging out of all of these examples we're seeing in that out there in the market is there's definitely lesser emphasis on accuracy and there's more emphasis on applications of things that are less, where the downside is not crazy really. That's really what it comes down to, right? Where the risk or the liability or the downside to using something like this is not a big deal, and you can play with it, and it's okay to be wrong. And it's more fantastic to be exploring all of this rather than be perfectly accurate on scale.
CostaSwitching a little bit to just the challenges that we've had, we've been using generative AI since, I guess, very early mid-2016 or early 2017. Right? And we were using systems that.. the systems that we developed early on had some very interesting properties. Really exciting kind of in the same way where you would generate things that you had not necessarily thought of. And in fact, we thought at one point, “Oh, this might be a nice system to design new..” - This is in the fashion garment space - And we were thinking, “oh, maybe this will be something that can design some new garments”, because when it goes wrong, sometimes they look interesting, but it's the same thing most of the time when it went wrong, it was just wrong. Occasionally you had something that you wanted to save. Well, one of the things that we found very quickly in terms of a commercial product and having and having customers involved is that if they gave us a garment and we wanted to do something with that garment. So our typical use case would be to take a mannequin image of a particular garment and transfer it onto a model in some particular pose wearing that garment. Then, when a retailer wants to be selling that garment, there's no room for error if you have this if you have a dress that has buttons down the front. You can't suddenly decide that, oh, I'm going to put zippers down the front, you know, or I'm going to have no buttons, or I'm going to make a long sleeve into a short sleeve or a long dress into a short sleeve. That just doesn't work. It has to be the garment. Or it has no value whatsoever. And so in our space, the job has always been, how do you use the artificial intelligence aspects to be able to change the view of a particular garment and be able to change, let's say, the size? They may give us only one sample size for the garment, but you'd like to be able to show it on a diverse set of models, right? Then you have to have a fairly sophisticated level of AI to be able to change the shape of the garment in an appropriate way to look like the model is wearing it properly. But at the same time, not damaging the garment and not changing any of the features in the garment, and having it be exactly correct.
Ashwini AsokanAnd resolution and accuracy is almost like the other end of the spectrum for us, right? Like as we've been going through this. And I think that we are producing at a really high-resolution today because these are very high-end brands that are basically using this as part of their website to showcase their products. And the expectation is that the customer is going to click on that product, look at the fall of the clothing, look at how the sleeve is. It's all about style, right? So it's almost like the leeway for getting it wrong is very, very little. So in some ways, I mean, this version of what we've been doing, I mean, it's almost like the exact opposite of that, which is it's all about accuracy and it's all about resolution on scale, the polar opposite of what you're seeing out there. And I do want to compare and contrast this a little bit, which is what we're seeing out there in the market today, is all about the possibility of creation, the possibility of discovery, exploring. It's all about range than it is about resolution, right? And when you are talking about deploying production scale, generative AI for very large enterprises, you are talking about liability in terms of people making decisions, right, in terms of revenue, in terms of automation, and in terms of cost. And you're talking about things that you get it wrong. The implications are pretty wild, right? And so the use cases that you get from going this way versus that way, I think it's very important to almost just let that sink in that these are two exact opposite ends of the spectrum here.
Meet your speakers:
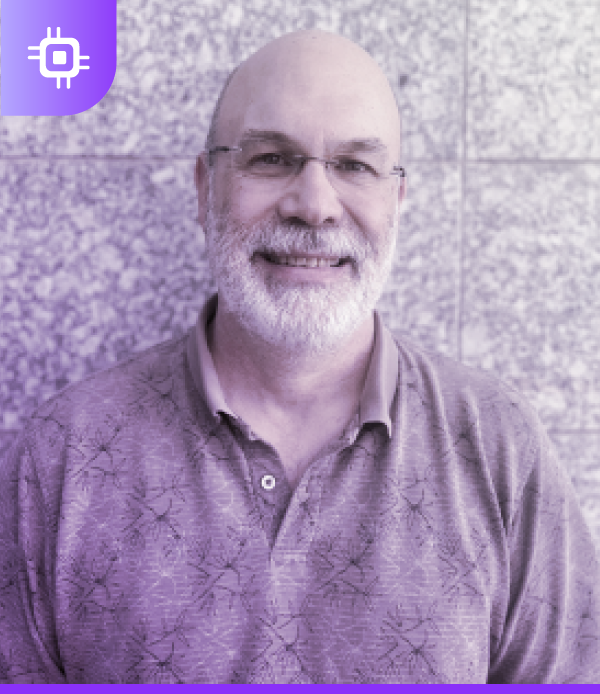
Costa Colbert
Co-founder & Chief Scientist, Mad Street Den
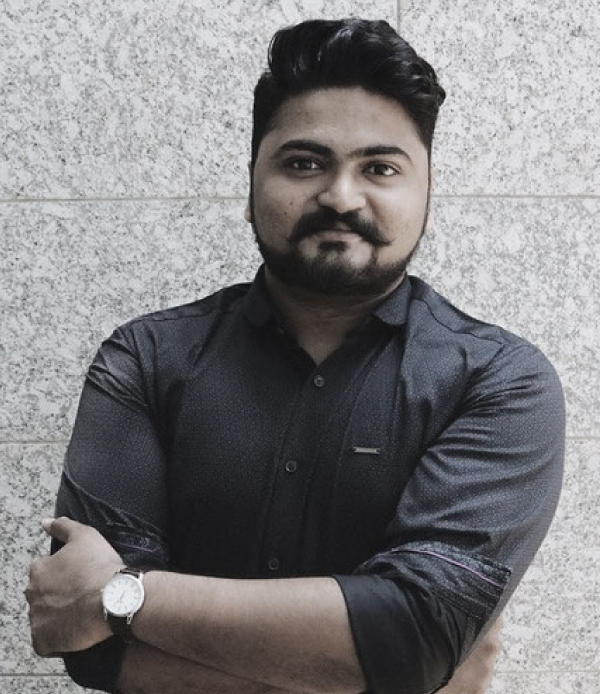
Shyam Ravishankar
Manager - Digital & Content Marketing
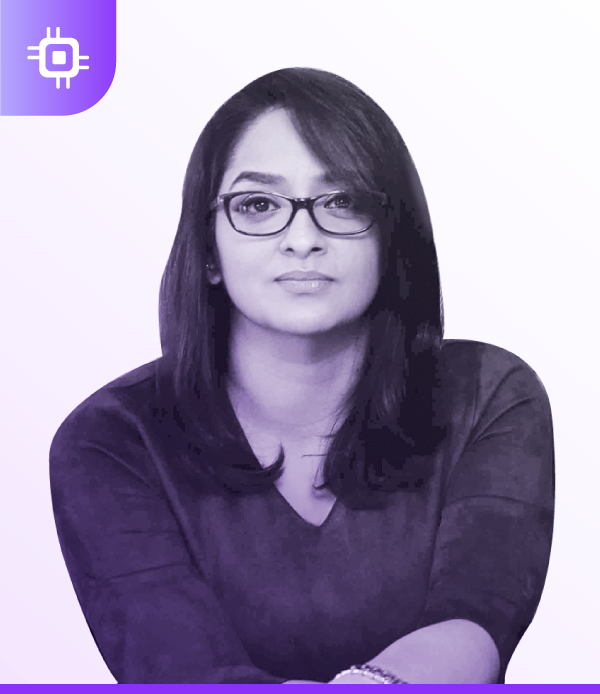
Ashwini Asokan
Founder & CEO, Vue.ai