Artificial Intelligence (AI) in Retail 2024 – 7 Real World Use Cases16 min read
Table of Contents
It is estimated that there are about 2 million fashion brands in the world – and growing. The retail industry is growing at an incredible pace and customers are finding themselves increasingly spoiled for choice. The flip side – brand loyalty and recall is dropping fast.
Customers are increasingly paring down their shopping to brands that connect with them, brands that offer personalized experiences, and talk to them on the channels that they are using.
Why every retailer should adapt to Artificial Intelligence (AI)?
The new generation of shoppers, the millennials, expect bespoke shopping experiences in real-time and at the lowest price. They expect the retailer to know them as well as their local grocer used to. They don’t want to be flooded with thousands of irrelevant products. Instead, they want shorter wait times, quicker order fulfilment, personalized product recommendations & frictionless checkout experiences. This new generation was brought up in a digital world where they can find multiple options with a click of a button. They are used to Amazon’s seamless shopping experience. This means retailers today have to adapt Artificial Intelligence (AI) to survive.
Research shows that when shopping experience is highly personalized, customers indicated that they were 110% more likely to add additional items to their baskets & 40% more likely to spend more than they had planned.
It is also increasingly clear that while retail is not exactly dead, it is undergoing a metamorphosis. There is still value in the offline. In fact, Doug Stephens, The Retail Prophet points out that stores need to see themselves as an extension of their media platform.
Are you on the right channel, at the right time?
The use case for the store is now to deliver a high level of experience – sensory and emotional in a way that is memorable and trust-building. But online is where sales are moving.
Approximately $5T of the $28T retail market is now online.
Retailers are finding their biggest competitors online. Social media platforms like Netflix, Instagram, Snapchat, and the likes have achieved the level of individualization and experiential content needed for a platform to become habit-forming – something that retail has fallen behind on. Shopping too is moving to these platforms.
And a large amount of this shopping is being driven by millennials and Gen Z.
It is easy to spot an item of interest on a platform, get recommendations, and opinions from friends and family, purchase the item of choice all within the same app and have the entire experience be tailored to the individual.
The data challenge
A presence online is not going to solve retail’s problems completely. Retail’s bigger issue is the lack of accurate data. Even the data that is available ends up being used ineffectively or in a way that doesn’t provide a singular view into the customer across channels.
Consider this – bad data has cost US businesses $3.1T every year, & data workers waste 50% of their time finding and correcting errors.
The challenge for brands in this atmosphere is two-fold:
1. Know the customer well.
2. Use the customer data and build operational efficiency into their systems.
Accurate data can bring optimization, automation, and competence. And the best way to use data to bring about this hyper-personalized experience is through Artificial Intelligence.
Artificial Intelligence(AI) in Retail 2024

AI is retail’s superpower. Retail is, in fact, one of the few industries where the adoption of Artificial Intelligence (AI) is being seen in a meaningful and actionable manner. Every step of the retail process has the ability to be automated by AI in a way that would increase accuracy, efficiency, and scaling of operations. From customer acquisition using reliable data to catalog and inventory management to post-purchase experience – AI has the ability to impact retail in a holistic end-to-end manner.
With Artificial Intelligence (AI), retailers can create data models to derive insights and in-turn build prescriptive or predictive decision engines. This can help retailers with things like demand forecasting allowing them to make better data-driven decisions.
Artificial Intelligence(AI) in Retail can also be used to structure and transform data from a semi-structured or an unstructured format to the one used by the marketplace. Algorithms can be used to identify and transform attributes from tables of data or even paragraphs of text
Here are a few use cases of Artificial Intelligence(AI) in Retail.
7 Use Cases of Artificial Intelligence (AI) in Retail
1. Data creation and labeling
The problem of inaccurate and inconsistent data causing AI to take bad decisions can itself be solved by AI. AI-powered data creation and labelling happen right at the start of the product digitization process.
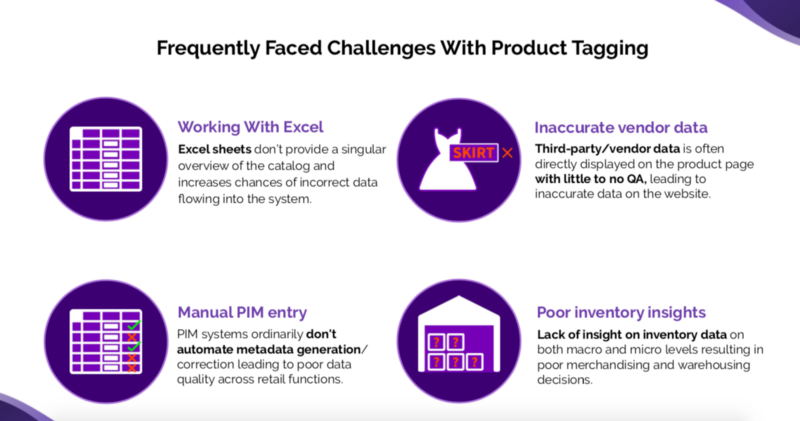
Artificial Intelligence(AI) can help in creating rich metadata for each and every product, eliminating human fatigue and errors. This can be done using Computer Vision algorithms which can automatically identify various attributes of a product and tag them accurately. The same algorithm can also be used to generate titles and descriptions for the product resulting in SEO-ready rich metadata which is easily searchable.
Reliable, SEO-ready tags, titles and descriptions can considerably boost product discoverability on search engines like Google and Bing. They enhance the metadata of the catalog, augmenting the quality of information available on each product. This metadata will also be indexed by search engines, and in turn, boost the ranking of the product in the SERP. When this metadata is combined with customer ratings and reviews, the product can reach the very top of the SERP resulting in an instant boost to the sales of the product.
Vue.ai uses Machine Learning (ML) to comprehend the taxonomy of each and every outfit, train the machine using in-house neural networks, which it then uses to generate tags for the outfit. Automated product tagging gives more accurate attributes for products and helps in better search results.
Image recognition driven product digitization produces above 90% accuracy and a more than 20% conversion through better search, browse and SEO.
2. Data Structuring and Transformation
Data, once created or extracted from the manufacturer’s catalog, now needs to be structured and transformed so that it remains consistent across several products, product types, and brands. A marketplace that sells products from hundreds of brands would find it extremely difficult to manually structure data.
For example, brand A might call the color of a dress orange and brand B might call it tangerine sunset. While searching for orange dresses, brand B’s dress will not be shown resulting in low discoverability. Now imagine this problem across hundreds of thousands of products across attributes like size, color, neckline, dress length, sleeve length, pattern, etc. If a marketplace sells products across categories like fashion, electronics, and furniture, this can get even messier resulting in irrelevant search results that directly impact the revenue of the marketplace.
Artificial Intelligence(AI) in Retail can be used to structure and transform data from a semi-structured or an unstructured format to the one used by the marketplace. Algorithms can be used to identify and transform attributes from tables of data or even paragraphs of text. Once it is trained with enough data, patterns can be identified and auto-suggestion of attributes can be used to quickly digitize products across brands and categories. This can help in cleaning up, grouping, categorizing, searching, sorting, and filtering data on the marketplace.
3. Data Insights to drive better decisions
The data that has been created, labelled and structured can now be analyzed to identify trends and patterns that can help make better decisions. Exploratory analytics is the most basic thing that can be done with the data to identify trends like “the revenue in the top-wear segment went up by 23% this month”. While this is fairly simple, this kind of analytics cannot be used to automate decision-making engines.
With Artificial Intelligence(AI), retailers can create data models to derive insights and in-turn build prescriptive or predictive decision engines. This can help retailers with things like demand forecasting allowing them to make better data-driven decisions. With time, these predictive models can learn to make better decisions taking more and more data into consideration allowing the retailer to accurately forecast trends and be prepared for them.
4. Product Discovery
Product discovery is the first, and most crucial step in a shopper’s journey. A simple and straightforward discovery process can endear the retailer to the shopper. A shopper who finds exactly what they are looking for is way more likely to come back to the same retailer for their next purchase. On a website, discovery begins with the site personalized search.
59% of web visitors frequently use the internal search engine to navigate on a website. – Neil Patel
Search engine users are high intent shoppers and represent a crucial segment of any e-commerce site. The results of a search on a website need to capture the intent of the query and show products that match the search term as close as possible.
With accurate, consistent, and structured data, the problem is no longer about the relevance of the results to the search but more about the relevance of the results to each individual. In other words, are the search results personalized to each individual’s style preferences?
For example, given the search term below “Wine Ralph Lauren Off Shoulder Evening Dress”, it is important to make a clear distinction in the search results for Becky and Lisa, given that Becky has a greater affinity towards the brand and Lisa that prefers having dresses that are closer to her color preference.
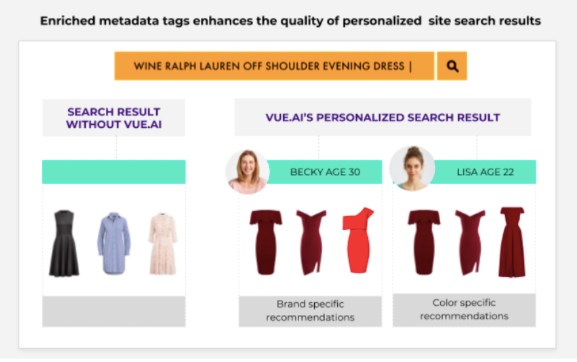
As seen above, an AI-powered Retail personalization search in retail can help tailor the search results, by boosting specific affinities for each shopper. Each click can be used to learn about the shopper’s preferences and converge to a hyper-relevant search bar that understands the customer.
Product Visualization /Visual Merchandising
Fashion websites need to stop using flat lay images and ghost mannequins for product visualization. When shoppers see products on models, they are able to visualize them better. It is even better when shoppers can see dresses draped on models of various sizes and ethnicities which can help depict The website and the products become more relevant to them and they are able to relate better. This leads them to browse and engage more.
A large chunk of eCommerce returns today happens because shoppers feel the product looks different in person. Retailers can help shoppers understand products better by showcasing them on models they can relate to, in realistic sizes similar to their own.
This means retailers need to show the same garment on
– Models of different ethnicities
– Models standing in various poses
– Models of different sizes
Retailers across the globe spend approximately $100 to $1000 per product on photography and digitization. The high cost of product visualization manually also means the merchandise is more likely to be non-inclusive and fails to give an accurate picture of the product. The time-to-market is also prohibitively slow and expensive.
Instead, retailers can use AI to generate automated on-model fashion imagery using Generative Adversarial Networks (GANs) and drape the same dress on models of various sizes and ethnicities standing in different poses without having to photograph all of this manually. This can reduce photoshoot costs, and speed up go-to-market while at the same time, increasing shopper engagement and conversions, and reducing returns.

5. Real-time personalization of the shopper journey
In 2022, brands need to know the shopper as well as the shopper knows the brand. Brands need to understand the visual preferences and style of each shopper to tailor journeys exclusively for each shopper. Brands need to create a style profile for each shopper to personalize content, offers, and products across all channels. But what is a style profile?
Style is a combination of visual and non-visual preferences like colors, budget, patterns, sleeve length, collar type, brand, etc. A shopper is far more likely to buy if the products are close to their personal visual style. No two people look at a product in the same way. Each shopper has their own unique style that is bound to influence their decision to choose a preferred website to shop on. It is possible to glean the style profile of each shopper not only from their purchase history but from each click on the website.
With real-time personalization, retailers can understand shopper intent with each click and serve personalized product recommendations with the highest likelihood of engagement.
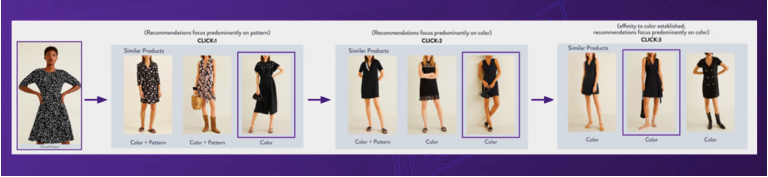
6. Personalized Curation
Shoppers in 2022 don’t want page after page of irrelevant products. Instead, they are looking for unique collections curated specifically with their tastes in mind. They expect stylists to personalize the whole look according to their own style profile. However, personalizing hundreds of looks for each shopper will need a whole army of stylists toiling away for days.
An AI styling assistant can curate looks, mood boards, outfits, and collections for each shopper based on their visual style preferences. Each shopper gets personalized styling recommendations for every product they browse, increasing the number of items in the cart while at the same time giving a great experience to the shopper.
7. Cart Abandonment Emails
Shopping cart abandonment is when a high-intent shopper visits an ecommerce website, adds at least one product to the shopping cart and proceeds to exit the website without completing the purchase. Retailers were reported to have lost a whopping $4.6 trillion to abandoned merchandise in online shopping carts according to Business Insider.
While retailers can do simple fixes to prevent cart abandonment like decreasing the load time, optimizing the website for mobile devices, keeping checkouts short and simple, keeping all pricings transparent, cart abandonment is still bound to happen.
Cart recovery emails are used by more than 62% of retailers around the world and are a proven way to recover carts. Nearly half of all abandoned cart emails are opened and over a third of clicks lead to purchases back on site according to Shopify.
But shoppers get emails from multiple websites every single day. How can retailers make their mails stand out?
An AI-powered cart recovery tool that recommends visually similar products and styling suggestions personalized by understanding each shopper’s visual style profile can power compelling cart abandonment emails that make cart recovery a habit. These emails add value to shoppers and one open can lead to shoppers opening every subsequent email as the content is completely personalized. This not only recovers the cart but also tells the shopper that the retailer understands them.

Conclusion
Artificial Intelligence(AI) in Retail can help teams across the globe improve efficiencies across their workflows. Automation processes help brands with accurate data creation and help provide context around shoppers’ needs and behaviours.
By getting access to this accurate data and using it across personalization, styling, and customized marketing, brands can leverage this information to curate experiences that are meaningful and valuable to the shopper.
Today, intelligent retail automation is about leveraging the power of data. The amount of data that needs to be processed from even a single shopper with several touchpoints across multiple channels makes automation a necessity, not a luxury. Failure to automate can even lead to retailers missing out on capturing data completely. And in a data-driven economy, this can have dire consequences.
There is a constant need to create, use, analyze and distribute data in a timely manner for instant contextual decision making and value creation. This can only be done with automated systems capturing data across different touchpoints and channels.
Retailers today not only need automation to capture data across touchpoints and channels but also need to unify this data to speak in a single voice to the shopper. A shopper should be able to transition seamlessly from online to offline and back online again. This means, the data captured on a website should be available to the sales assistants in-store and the products bought by a customer in-store should be used to improve the recommendations on the website. Data cannot be isolated in silos. It needs to flow both ways and help provide a complete story about each shopper.
With the power of data, intelligent retail automation can completely transform how retail teams function. Automation can completely take care of efficiency, giving retail teams time to think creatively and provide incredible experiences both online and in-store.
When retail truly becomes efficient and experiential, customers can enjoy the thrill and the magic of shopping anywhere, anytime.
General FAQ
Every step of the retail process has the ability to be automated by AI in a way that would increase accuracy, efficiency, and scaling of operations. From customer acquisition using reliable data to catalog and inventory management to post-purchase experience – AI has the ability to impact retail in a holistic end-to-end manner. With Artificial Intelligence (AI), retailers can create data models to derive insights and in-turn build prescriptive or predictive decision engines. This can help retailers with things like demand forecasting allowing them to make better data-driven decisions.
Here are the most important AI use cases in retail.
1. Data creation and labelling
2. Data Structuring and Transformation
3. Data Insights to drive better decisions
4. Improve Product Discovery
5. Product Visualization /Visual Merchandising
6. Real-time personalization (personalization engine) of the shopper journey
7. Personalized Curation
8. Personalized Cart Abandonment Emails