Two Founding CTOs Talk Tech, Data and Teams6 min read
Table of Contents
We recently hosted REBUILD – a transformative experience that enables trailblazers across categories to come together and lead the AI-Native movement.
At the event, we were thrilled to have Sauvik Banerjee, Founding CTO, Tata Digital and Anand Chandrasekaran, Co-founder, Blox.ai. They delved deep into the nuances of building and scaling tech teams from 0 to 1. They also emphasised on why data is the singular most important thing for an organization today.
“We drew up our core pillars for technology and platforms. We decided we had to be super resilient, very scalable, Agile-native and cloud-native. We are also fundamentally thumb-obsession first. Apart from this, we are automation first and we are extremely sensitive and secured as set of platforms,” Sauvik said on the core tenets around which they’ve built their tech stack.
Here’s what they had to say on all things tech, data and teams –
Go cloud-native first
“The concept of the Tata Neu ecosystem was there in virtual PPT.com. But that was just the germination. On Day One, we made sure we were on the cloud and were able to spin up an environment before the concept,” says Sauvik.
When tech leaders set out to build their stack in this digital age, taking advantage of the scale, elasticity and resilience that the cloud provides is their best bet.
With technologies such as Kubernetes, Docker, serverless functions and APIs, developers can reduce operational tasks and build applications faster. They can also scale capacity based on demand, without the risks associated with in-house operations and maintenance.
Being cloud-native gives teams the ability to spin up new computing instances within seconds and design architecture. They can also test ideas without the dependency on on-site hardware limitations or slow procurement processes.
“We were cloud-native from Day One,” said Sauvik.
Tackle the build vs buy conundrum
When it comes to software, businesses regularly struggle with the question of building vs buying. On one hand, a purpose-built solution might be the best way to tackle a use case by meeting exact requirements. On the other, off-shelf solutions could reduce costs related to personnel requirements, maintenance, and time to value.
“We didn’t want to build certain things because there were too many things that were already built. We decided that we would keep our core principle of homegrown versus COTS or out-of-the-box products. We said, we’ll keep 70% of it homegrown and 30% of it could be enterprise COTS products,” said Sauvik.
Today most organizations approach this in one of the following ways – build, buy, or the hybrid route.
The build route is the way to go for businesses that are lush with funds to experiment. It also takes at least 2 years to see outcomes from the investment. Whereas with the buy route, organizations can gain an advantage by getting their engineers to work on quick wins with ready-to-use APIs. This means they can focus on immediate ROI.
The hybrid approach combines the best of the build & buy models with a laser-sharp focus on ROI.
Ultimately, it depends on the value that organizations wish to create with their stack and the ROI that they expect. Balancing immediate needs with long-term vision will determine the right decision for each business.
“We went for an enterprise stack. And wherever we have done so, our principle was we will jointly build IP. That’s something which we have treasured as a principal of this organization. The success is jointly owned, the risk is jointly owned, and the failure will also be jointly owned,” notes Sauvik.
Fix your data
There are no two ways about it – today data everywhere is ugly. Point solutions fall short of achieving their goals simply because models are only as good as the data they’re built on. This data is generally inconsistent, incomplete and siloed.
“What we have done well as a team, I think we have identified certain signals and we wanted to do that in the first phase of our launch for the last 18 months. And the data was ugly. Data was ugly from historical data and data is ugly everywhere we are,” said Sauvik.
Consumer data typically comes from so many sources that it is often siloed and lacks a single source of truth. As a result, insights drawn from these disparate datasets are inaccurate as well. By consolidating transactional and behavioral signals across various channels and standardizing them in formats that are compatible with internal workflows, businesses can feed actionable insights to downstream processes. They can leverage their foundation of good data to act as a launchpad for sensemaking applications such as prediction, personalization, targeting, and more.
“We are the Pied Pipers who are supposed to clean it up,” notes Sauvik.
Transform your data meaningfully to create your biggest differentiator
“The world has been obsessed with data. Yes, we are collecting a ton of data. We all know that we’re going to figure out how to use that. But the middle ground between ‘getting all the data of the world’ and ‘actually doing something useful with it’ is missing,” says Anand.
In order to effectively consume AI, it’s important to understand the lifecycle of data.
The data that sits in warehouses and data lakes is a result of the interactions that people have had with it and is a reflection of the journeys of the people who have created it. And in order for these journeys to make sense, they need to be viewed in various modes until all the different pieces flow together. In other words, in order to actually make sense of data, it needs to be processed, organized and contextualized in a meaningful manner. Unless that’s done, the data is going to be useless by itself. On one hand, teams are heavily investing in collecting data, and on the other, they know exactly what they want from their data. But in order to actually bridge the gap between the two, the data needs to be meaningfully transformed into understandable domains that can then feed into the analysis.
“This is this is very similar to how a neural network is built,” says Anand. A deep learning network was built on the fact that if you had a data manifold, you could not piece it apart and say this equals that. You need to transform the data, see it from a different angle, and transform it again through another layer, another layer, another layer, until you can make a simple choice to say, ‘Do this for this person or ‘do that for that person.”
Invest in great talent
A business is nothing without the people who build it. Sauvik’s advice for budding CTOs?
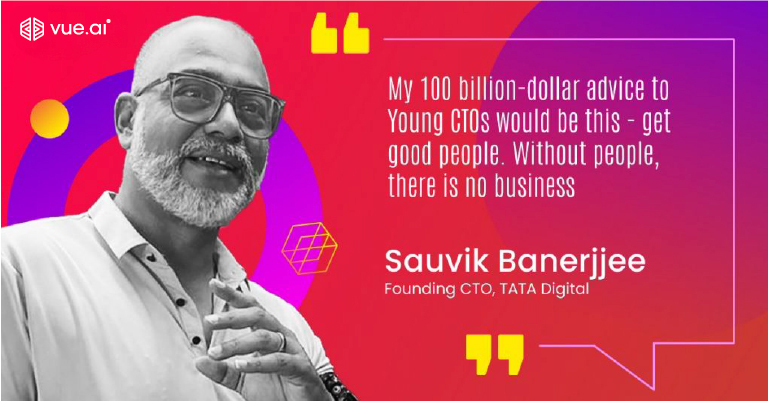
Before all else, the founding team at Tata Digital prioritised building an all-star team to bring their vision to life and scaled their teams from 2-3 to more than 20! “We went into campus and we started recruiting the young Gen-X brilliance, which is the fulcrum of Tata Digital,” he says.
Today, six months since they set off a their journey to build the country’s first super app, the Tata Digital team is 100 members strong and still growing!
“That journey is very interesting, but the people-first architecture was the crux of it,” notes Sauvik.