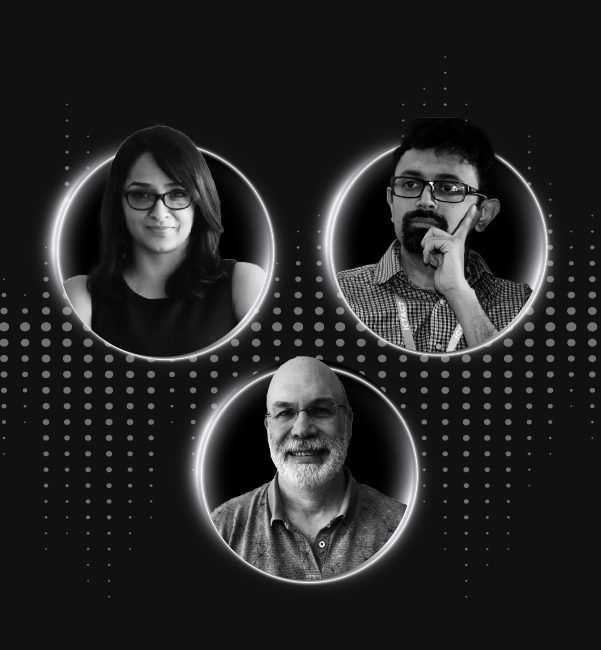
From Noise to Signal: A 2024 AI Memo from Ashwini Asokan, Anand Chandrasekaran and Costa Colbert, Founders, Vue.ai
About this Podcast:
This episode of the AI Transformation Podcast featuring Anand Chandrasekaran, Ashwini Asokan and Costa Colbert, founders of Vue.ai unveils a new branding for their company that accentuates the theme of ‘uncomplicate’. The founders discuss their journey, the evolving AI space, and the launch of an AI orchestration platform. Ashwini highlights the culmination of years of AI discussions and the challenge of navigating an overwhelming, jargon-filled AI landscape. Costa emphasizes the need for a platform that seamlessly integrates traditional computing concepts and AI, avoiding the chaos of piecemeal solutions. Anand discusses the fundamental shift in people’s thinking towards adopting new technology and the importance of orchestrating skills and data for effective problem-solving. The founders stress the platform’s composability, orchestration, and automation, envisioning it as an AI-based operating system for enterprises, simplifying the complexity of AI adoption and delivering concrete outcomes.
Listen on:
Episode Transcript:
Hey there! Welcome to the AI Transformation Podcast by Team Vue.ai. I'm Shyam and I host this podcast along with my founder, Ashwini Asokan. We've been bringing together leaders from across the world CIOs, COOs, digital transformation heads, CTOs, product and marketing leaders who have been crucial to transforming their businesses with AI. This episode is going to be a special one featuring our founders Ashwini Asokan, Anand Chandrasekaran, and Costa Colbert. They're going to be talking about AI in 2024. Our journey till now, what's up ahead and our brand new AI orchestration platform. Over to you, Ashwini.
Ashwini AsokanWell, okay, we're finally here, and it's time for the big rollout. Very exciting to kind of be at this point in time, at this moment, for the three of us as we launch this new branding and with a very, very important tagline, if I may add,uncomplicate. And with this new branding, we're basically revealing the story of our platform and what's to come in the next 12 to 18 months for all our customers and the different industries that we work with. For me personally, and I'm sure this is true for the two of you as well, it feels like the culmination of of years of ideas about how to build AI, how not to build AI, just conversations that we've been having with people that have been trying to adopt AI on scale inside their organizations. It feels like the culmination of a lot of kind of hypothesis around. What's the right way to put something out there that allows companies everywhere to adopt all of this. Towards very clear, concrete outcomes, right? Rather than just be lost in this jargony you know what's new? What's new? Kind of hyper world that we're in with AI?
Costa ColbertYeah, exactly. Exactly. I think one of the, one of the things going way back to when we started talking about the idea of having of having a general AI platform. Of course, we were thinking as much about generalized intelligence, but at the same time thinking about the breadth of things that we could do and not only doing things very, very piecemeal and, how to put together a system that can do whatever all comers, you know, want to be able to do. I think the other part of it is that if you think about the last 5 or 6 years, AI has basically been attached because of marketing. It's been attached to basically anything a computer could do. It used to be anything a computer could do wasn't AI, and now all of a sudden it became anything a computer can do must be AI, right? And so kind of reverse. So having a platform where we don't really need to make the distinction between whether or not something is AI or not, even in the cases when it really is, you know, is something that we've really been shooting for to be able to very nicely and seamlessly meld together traditional computing concepts, along with new new technologies and artificial intelligence that come along. So you're not just ripping out the, you know, the old and trying to stick in a new, but you can you can kind of meld in with a tried and true way of doing things, which is what most companies still have, and most companies still have teams that have different levels of expertise, and they still need a lot of help for all these different things. And now we're finally in a position where we can start offering something that can that can serve people at different level with different, different levels of expertise in their teams and the like.
Anand ChandrasekaranJust to add to that, picking on adoption of new technology, given there's a lot of new technology, particularly this year, that's that's looming in terms of everybody trying to adopt that latest technology to solve every one of their problems. It's the,there's this fundamental shift in how people are thinking. And I think it's, , it's funny. It's you want to solve all your problems. And so you are trying to load each in every solution with everything, the kitchen sink. And that's like saying, if you translate that into the human world, it's to say that you employee X, you have to be greater statistics, greater computer programming, you have to play football, you have to be an astronaut. You also have to be an artist, and you need to be a good father or mother and everything at the same time all at once. Right.
Costa ColbertThat's right. For that entry level position.
Anand ChandrasekaranYou need to have ingested the sum total of all of mankind's knowledge. To be an entry level. Obviously I'm exaggerating a little, but that's the situation we seem to be finding ourselves in.
Ashwini AsokanAnd I think, you know, that that whole entry level, I particularly love that comment. Right. Because I think on the other side of it, if you if you think about what the teams that are faced with these kind of decisions, what they're going through, right. And, you know, we've been sitting on, what, seven, ten years of data from talking to all of these folks that are actively trying to do all of this and roll out all of this inside of their organizations. There's a little bit of which way do you go? There's so much. It's just noise. It's noise everywhere. Right. And it's and you have this explosion of applications, explosion of infrastructure and and inside all things infrastructure. There's like a line item for that, right? Like every little line item in your data stack has ten components right now. Right. And I think it's ended up becoming an architecture nightmare, right. More than anything else, it feels like that's the issue. Which is which part do I get? What do I put in there? And if I make that decision now, how is it going to affect me like one year later when someone else wants to build another application? Essentially, what what am I going to do with this? Right. You got to know everything as well, right? So there's all this expectation around the AI itself, and then there's expectation around the teams that are putting this all together to kind of get it right. Right. And the hardest part about getting it right, I think there are three, three kind of themes we hear from everybody we talk to about, you know, what's wrong with that kind of expectation. Right. And the first is. It takes 4 to 5 years today, right? Like it's a transformation project. Right. And we think I think the industry has tried just about everything else. Digital transformation, you know, AI transformation just about everything. And it's and it's a five year playbook. And the biggest conversation there is no like the attrition in organizations are massive. Right. Like the the average I think tenure of a chief data officer in most organizations is 18 months, right. The average, you know, data scientist or ML or analytics person in most of these organizations is between 18 and 24 months. And all of a sudden, you know, there was a particular customer in a recent sales call that kind of joked saying, you know, at the end of a four year, you know, project, the only people left at the table were E&Y. Yeah, like nobody in the company. Right. Like it was the consulting teams that were basically left and just nobody else. And all of a sudden, you know, it's not just the expectation around the AI. It's just like who's making these decisions, who's owning it? And what's that going to look like for the longevity of the company and the things it needs to get done. And that's that's beginning to turn out to be a nightmare?
Costa ColbertYeah, I think so. I think so and I think part of what people forget is that just because you have a solution to a very narrow problem, does not a product make and does not a solution for a product make, it takes a ton of infrastructure. It takes a ton of knowhow in, you know, going on an analogy there. In fact, you can't have a single person know enough about every one of the of the different pieces. And so you end up having to, have that knowledge distributed amongst different people. And when those people turn over, the next guy that comes in, first of all, has to be able to jump in and take over. But you also have using sort of legacy systems and doing things piecemeal the way it's been done. You know, one thing from one vendor, another thing from another vendor, a third thing from a third vendor. So first you have the initial difficulty of connecting all those pieces. But what's even worse, I think, is that you put together a system, maybe it works great. And then, you know, maybe you lose a couple of the people that worked on it. Maybe. Maybe you don't. But you go on to another project. Years have changed. A couple of those pieces are not relevant anymore, or the next best thing has come out.So you want to be able to change it. And before you know it, you have all of these different systems within your company that are built on different technologies. And now all of a sudden you have this multiplication of people having to have expertise, not to solve one problem. But now, you know, do I know the best about every different database and every different caching system and every different, you know, gooey system? What's the latest web technology and all of these, these different sorts of things. And so getting back to this idea about the platform itself having a structured way that handles the different pieces of this, you know, allows you to go in and solve, solve these kind of problems. You can have somebody come in and know how to work. They work on the first project, then they work on the next project, and they bring over the expertise that they've had because they're not having to use multiple different vendors tools and they're not having to, you know, and there's enough abstraction within in the system that if you do have to have a database get changed out, it doesn't mean that everybody has to be an expert in that, because it's already been abstracted a little bit.
Ashwini AsokanYou know, the industry, two particular terms here. That one is the composability aspect, right? I think that's a key part of what we're talking about here, which is, you know, I think we are in the era of composable systems, and we have adopted that in this new category, which, you know, now might be a great time. To kind of bring that up is we're calling this the orchestration category, right, as opposed to which is pretty much reflecting what you're saying here. Costa. Right. Which is how do you build these composable systems that allow you to put in take out, right, and allow it to kind of almost morph over the over the kind of lifetime of that company as it needs as its needs kind of change inside the organization. And so the composability is one aspect of it. And the other aspect of it, of course, is being the orchestration. Right? Which is I is not so much anymore about just there's an app for that. I keep saying this right. Like,we've it felt like the last five, six years was like that Apple ad, right. Like where you just ended up, you know, stumbling into the AI industry. And there's an app for that. Right. And and there's an AI, you know, agent for that, or there's an AI kind of automation for that. And I think we're going from that era to the era of composable systems, which you have to have AI to essentially orchestrate between those systems, like manage all that data, talk across everything. So it feels like the role of AI is less so about kind of, oh, I can do that too. More about how do you orchestrate between all of that?
Costa ColbertYeah, yeah. How do you get how do you get your product put together that you need?
Anand ChandrasekaranIf I'm going to use a story I'm obviously going to obfuscate some of. Details out of sensitivity to who I'm talking about. So we encountered this client, um, who had a particular problem that they needed to solve. And, and this is something that happens in most of these calls, which is our data scientists took the data we had, put it in SageMaker and got a model out of it, and the model was performing pretty great, 85%. And then when we deployed it, we got 30% in the field and we don't know why. And when you dig a little deeper, you understand that there are certain fundamental flaws to this approach. You know, you can't just build a model with the amount of data you have, and it won't survive the real world, because there are a couple of problems here in this particular case. The amount of data fed in was low, but that's not like a silver bullet. Feeding more data is not the problem in this particular case. The way the data was collected had certain fundamental problems. The images were rotated in every direction that you want. It was an image classification type problem. But the way the way the data was collected, there was no standardization in terms of how the images were. So what you have over here is. You took the data you had and you dumped it into a machine learning algorithm, and you train a model and it gave you a certain degree of accuracy. Now, what did that algorithm understand? It picked whatever was there in the data you fed in, and gave you an accuracy because it picked up on any signals.But as a human, you know that you need to rotate the images, and looking at the picture the same way matters for how you solve a problem. Now we have taken that for granted because as humans, the invariance problem is already in built into solving mean. You rotate your head and look at me. You still know it's me and you take that for granted. But a machine doesn't have that solved it. Solving multiple problems to reduce the problem. To just tell me the difference between these two pieces of data, it's actually orchestrating multiple different skill sets, right? Which is did I rotate it the right way? Did I remove clutter? Did I normalize it? Did I make sure the lighting was the same? Is the zoom level the same on how I'm looking at this images? And then can I spot the difference between this? Now all of this is compute power. Right now there are two ways you can solve this. You can throw the kitchen sink at it, which means you feed every variant of every thing that is there on this planet. And yes, you can build a large model that will solve this problem regardless of how you took the picture. Or you could just use the fact that why don't you standardize how you take the picture? And then a very tiny model can solve this problem. So orchestration is not only about orchestration is also about our own intelligence and basic algorithms. And putting it together, you need to assemble a whole bunch of simple pieces so that the final problem that you're trying to solve has been simplified.
Ashwini AsokanAnd how you make it automated. Right? Because at the end of the day, I mean, you could do orchestration as a service, which is a nightmare because then you're completely depending on, you know, human inconsistencies, time, you know, different people, different types of skill sets. You're completely different, as opposed to automating everything that you just said, which is basically the principle with which we're launching this platform. Right. Like, you do not have to make all of these decisions. A lot of these things are automated in the form. And this might be a great time to talk about the federated engine approach. Right. And the contextual search, because those two become kind of essential parts to the platform that allow for this automation of the orchestration.
Anand ChandrasekaranYeah. If you had a whole bunch of skills, but at any point in time you only need a few skills to solve a problem. And obviously in the in the world of AI, we're saying there is a subset of the world's set of models that will solve your problem when you connect them together, the right way to solve it, as opposed to having the most powerful model solving even the most trivial problems. Right? So instead of trying to build one large model that solves every problems, if you could orchestrate and have a system automatically pick which skill sets to recruit to solve the problem at hand, then you can do this at a fraction of the cost and do it in a much more robust manner. And going back to your composability argument, you could build specialist models that are not useless.
Ashwini AsokanYeah, yeah. And the cost base I mean hello I mean it feels like that's the number one, this whole idea that the total cost of operation for adopting AI and for, for, for building this modern data stack is mean. Everything is a hidden cost. Everything is a hidden cost, right? You think you're buying something and then like, you're basically giving away an arm and a leg and, and half the company's revenue towards, you know, this and think there is this is probably on the list of, you know, problems that people talk about. This is probably like second or third, right? One is just the sheer overwhelming nature of like so many choices and architectures that you just have to constantly maintain through changing teams. And the second part of it is this, right? Like you're you're spending so much money across things and different people are accountable for different things, right? So you have the personalization guy come in and say, that's not my problem. The data is not clean. That's not my issue. And then the the data guy comes in and says, my job is to store the data. I'm not cleaning your data. And then the MDM guy comes in, the PIM guy comes in, right? Like the the warehouse comes in. And then all of a sudden the metadata management guy comes in. Who owns what? Like who's owning this, right? Like like who is accountable? And basically it's like that Spider-Man meme, right? Like everybody pointing fingers at each other. And at the end of the day, you're, you're you're you're kind of stuck with, with the cost and the bill without knowing what to do. Right. And so, I mean, I think the cost is a really, really crucial one. Costa?
Costa ColbertYeah. Yeah. And I think there's a, I think there's an under-appreciation too, for the idea that you can for, for most one thing that we've, we've learned quite a bit over the last few years is that there are different levels of problems in terms of what's an acceptable level of, of, let's say, accurate. See for a particular inaccuracy can mean a lot of different things. But you know, there's going to be some level that's good enough to for some particular use case. Right. And I think there's an under appreciation for, um, how it's actually fairly hard even what seems like a fairly easy bar, that it's actually hard to get something that works, especially if you're looking for scale and automation, something where you don't have to actually have people with their eyes on every particular thing. So we've had things before where you can you can generate something and you can look at it and you can get a few examples that look really good. You can use it as a proof of concept that something makes sense to do it a particular way, but for it to be a project, you know, for it to be a product rather than a project, then you it things sort of flip around. You start needing the majority of things to be right, and then things need to be much, much more robust. So even though there's a lot of times where in this AI space where you think, oh, I can just throw, you know, X at at this problem and it should be able to solve it. There's enough inconsistencies and enough of enough trouble with, with some of these systems that you really do need the structure of a traditional, you know, a traditional system. And to just be able to say, oh, I'll just take all my data. This is sort of what Anand was saying before that you can't just say, oh, here, I have a hard drive with 20TB of images, and I want to do something with it. Well, you know, maybe, maybe if you throw an automated system at it, it can handle it, but maybe you actually need to have some eyes on a lot of it before you realize that there's a lot in that data set that is not what you had intended, not in the least, you know, in minor ways or in major ways.
Ashwini AsokanAnd yet, I think you know and this is almost there's that point and then there's the point around somebody else wants to use the same data set for something else. Right. And so I think this is the other thing, which is everybody is mining the same data for completely different reasons across the organization. And I think one of the ways in which we've architected the orchestration platform as well is to say, hey, there are three types of people in the organization that will be using this, right? And one is IT guys who are basically managing large, large amount of business applications inside, and they need a lot of automation and across that, but more importantly, they are the source of all that data. Right. And so they're they're going to have to be kind of critical users of this. Second one is analytics teams because they're the ones who are constantly slicing and dicing the data and building reports and basically having a perspective on on where the business is heading. Right. Or all of those small parts inside the business is heading. And then there's the data science and ML teams who are actually, you know, automating it and building these models and much, much more richer models and kind of enabling business organizations to get the outcomes that they're looking for. Right. And, and in that zone, I think it's really important saying, okay, there's this data and this data. Everybody is looking at it through a different lens, which means everybody is expecting something different out of the same data. And then guess what? Everybody's adding to it. Everybody's removing from it. And you're like, what happened? Right. And it's changing. It's literally changing as the company itself is changing with time, because obviously a company is a living, growing, changing entity and the data is changing with it. And so all of a sudden, it's not just the I think one part of the issue is I'm looking at it, how do I deal with it? The other part of it is everybody is looking at it through a different lens, which means it's expected to do different things for different people and have different types of outcomes. And then the orchestration piece becomes that much more important through that. Through that. Yeah.
Costa ColbertThat's that's absolutely right. I mean, we had we had a particular example not that long ago where one of our, one of one of our teams was working with this company to solve a particular document processing problem. And it was actually fairly difficult. And so they were making their way through trying to understand how to approach this problem. And then just at lunch, you know, somebody just sort of mentioned, oh, you know, we're doing this. And somebody else at the table was, oh, could you possibly do that for us? You know, 5% different. It would make our lives so much better. So here they were struggling on this very difficult problem for one part of the company. And the other company could have made even much better use with a lower bar. But if they were relying on only their limited team, with so many people to be able to do this, they would have, you know, they would have had to scope out this, this whole project, realize, you know, realize what the differences are, because the data were not necessarily in a format that could be very quickly pulled out. And just use for this other problem, right? Exactly to your point.
Anand ChandrasekaranYeah so it's not just leveraging the right skills to solve a problem. It's also leveraging the right data right in. And often what happens is the best models that are built, they're working pretty fine are throwing away information. And in throwing away that information, because I'll just go back to the standard classification problem. Classified by very definition means that you're taking a ton of data and then you're putting a label on it. The machine is putting a label on it, and that label is supposed to capture everything in that data. Sure. For one particular application, based on how one person wanted to use it, or one team wanted to use it, but hidden there is that you have systematically thrown away information layer after layer inside that neural network or whatever you use. And somewhere there is information that somebody else can use. So it's not just federating from different models to solve the problem. Can you federate from amongst all of these layers of data that have been created in the process of solving a problem? And if you have that available for some other team to use, as Costa was just putting it it, you've short circuited a project that could have taken months to minutes, and there is no way humans can do that either, because now you're talking about the realm of data that has been abstracted, compressed, encrypted in some weird feature space, or embedding space and making that discoverable for different applications. So the orchestration platform has to not just pick the right skills to solve the problem at hand. It should also be able to query back into the system and say, oh, but this version of that data actually serves you better in solving this problem. So it's a combination of orchestrating the skills and the intermediate data sets, if you want to put it that way.
Costa ColbertYeah. You mean the silly example that pops into my head is remember the the hot dog not hot dog thing that somebody had done at some point. So just imagine you're doing you're doing, you know, hot dog not hot dog. But then somebody comes along and says, could you tell me where all the locations were by what was around the the hot dog, you know, is there a Eiffel Tower in the background or is there, you know, something, something going on like that? And so rather than having to say, oh, I could train a model for that, if I can track down the data again, that if it's in its original form, let's say, which was huge because there was a lot of that data. And in this case, you know, we can say, well, we might be able to go back and just mine some of this other information because at the at the early part of these neural networks, it's still a visual problem. And a lot of the features that have been generated are still visual features and the like.
Ashwini AsokanI think all of this broadly at some level, really just comes down to the fact that. You don't know what data is important, what's not. Nobody does. Right? What you may think is important is completely irrelevant to the next team. And what they think is important is completely irrelevant to you or.
Costa ColbertYou then, or you the next day really.
Ashwini AsokanOr you the next day. That's exactly right. Right. And I think this idea of and I, kind of push a little bit when I talk to folks about this to say, think of it as an AI based operating system, right. For enterprises, at some point, we genuinely believe that that's where we're headed as an organization. And with this kind of launch of orchestration, we genuinely believe we are embodying these principles around one on uncomplicating all the noise and the madness that this space has been with the idea of orchestration, which allows companies to basically take a very data centric data first approach to the kind of AI that they get to adopt and build inside their organizations while simultaneously taking a very outcome first right approach to whatever you do with the AI. Right. And this philosophy is almost at the at the core center of this rebranding, of this new launch of the platform. And, you know, we're going to take the next 12 to 14 months to slowly start creating this kind of rich canvas, we believe, for all of our customers to come in and paint with within their organizations and slowly roll this out over the next, like I said, 12 to 18 months.
Meet your speakers:
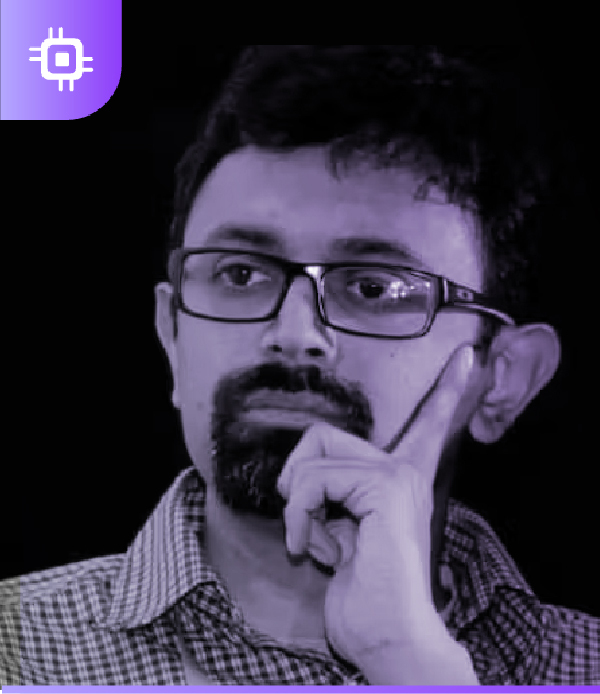
Anand Chandrasekaran
Founder and CTO, Vue.ai
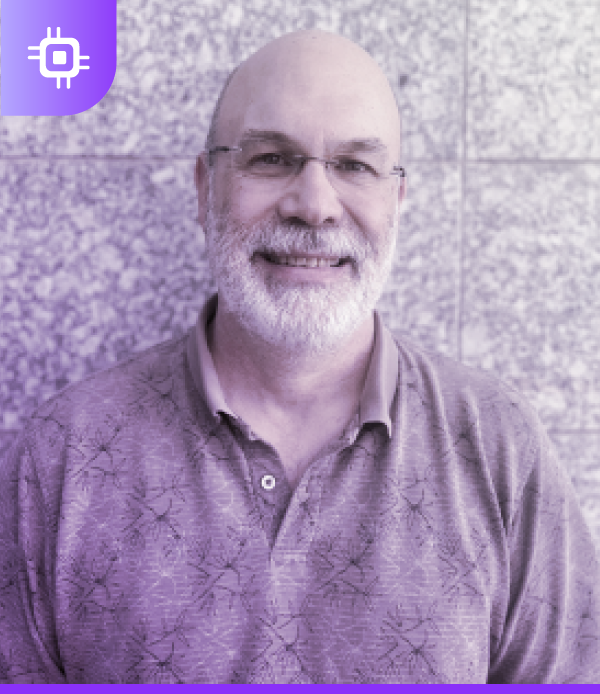
Costa Colbert
Co-founder & Chief Scientist, Mad Street Den
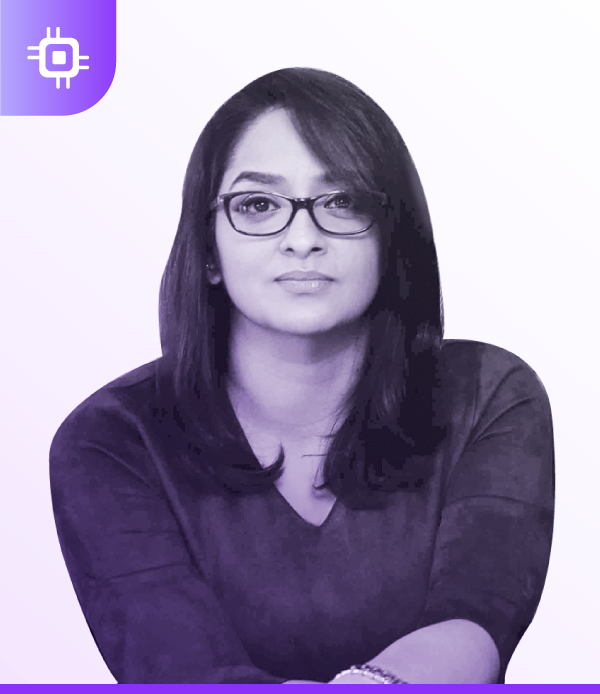
Ashwini Asokan
Founder & CEO, Vue.ai