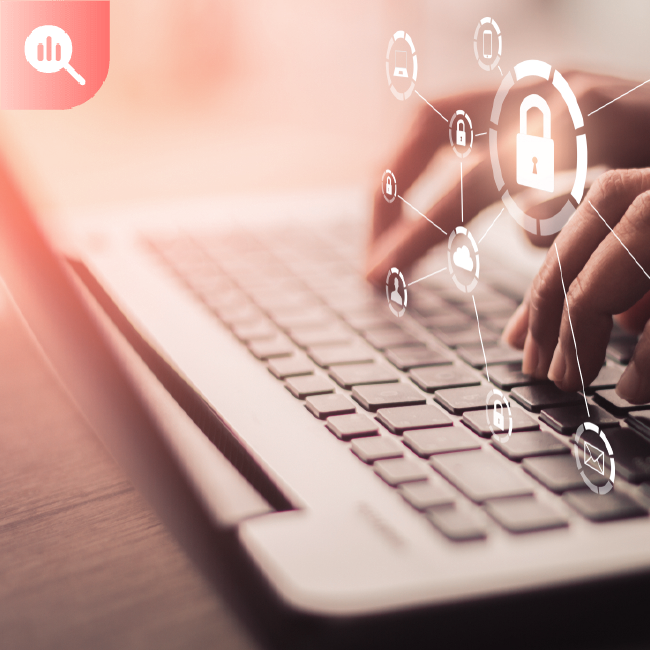
Data Governance and Groove with Tiankai Feng
About this Podcast:
Our guest today is bringing his expertise in data and passion for music to create an entirely unique learning experience. Tiankai Feng, Ambassador at DQ & Head of Marketing at DAMA Germany brings into this conversation, a wealth of knowledge and expertise in the field of data analytics, governance and compliance.
With over half a decade as a Senior Director of Product – Data Governance at Adidas, he delves into the various facets of data governance, the role of AI and his vision to make data accessible and fun for everyone working with it.
Episode Transcript:
Meet your speakers:
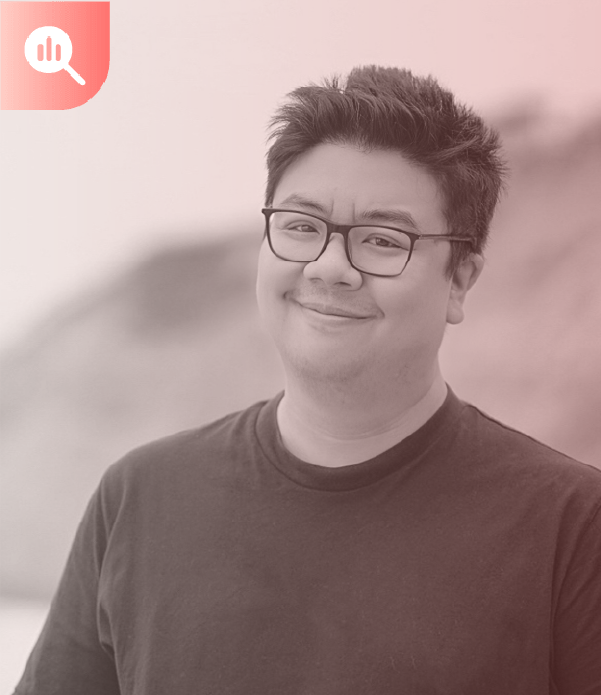
Tiankai Feng
Ambassador, Data GQ
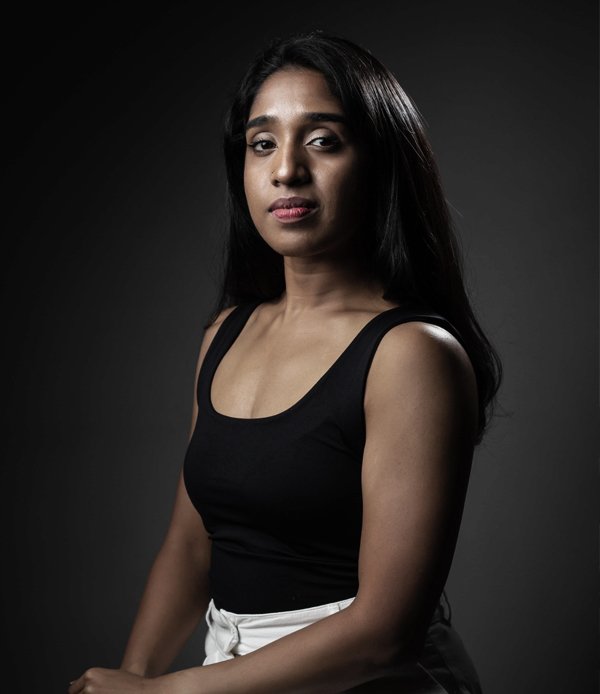
Krithika Anand
Customer Marketing, Vue.ai